AlphaFold: The AI That Solved a 50-Year-Old Protein Structure Problem
DeepMind’s latest version of AlphaFold can accurately predict a protein’s 3D structure based on its amino acids, opening new possibilities for fields from medicine to environmental science.
Reading Time: 4 minutes
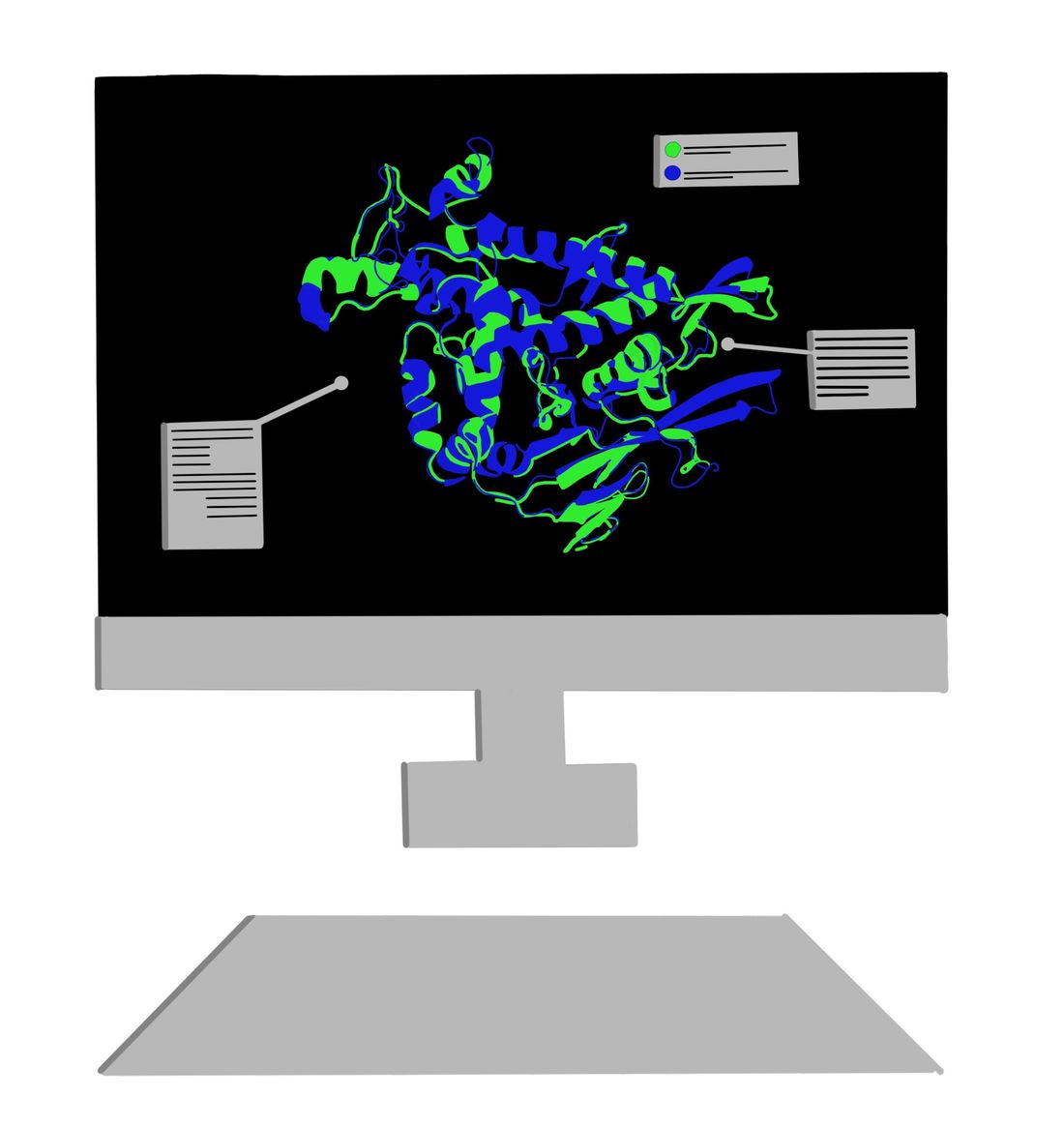
From your silky-smooth hair after taking a hot shower to the billions of antibodies circulating your body to help fight off infections, your complex body and its amazing capabilities are built by proteins, one of the fundamental building blocks of life. It is the proteins’ structural variety that allows them to perform so many different functions in the human body. So, it is no surprise that decades of research have been dedicated to developing technology capable of predicting a protein’s structure, as structure determines function. An AI network known as AlphaFold, developed by Google’s AI subsidiary DeepMind, was recognized in a competition known as Critical Assessment of protein Structure Prediction (CASP) for doing so with great accuracy in November 2020. With AlphaFold, scientists have gained the potential to not only achieve a much deeper understanding of protein structure and function but also utilize the power of proteins to revolutionize all fields of biology, ranging from medicine to environmental science.
The story of AlphaFold began in 1972 when Nobel Prize in Chemistry laureate Christian Anfinsen concluded his acceptance speech with a postulate stating that a protein’s structure could be determined solely by its amino acid sequence. Proteins are made up of molecules known as amino acids, and there are about 20 that occur naturally. As a protein typically has 100 amino acids in its sequence, an immense number of amino acid subunit combinations allow for the 3D structure formation of 200 billion known proteins worldwide. With such a vast number of proteins, technology that could easily predict a protein’s structure with just its amino sequence could cut down the time needed to understand each and every protein important to our everyday functioning. And while techniques such as X-ray crystallography, nuclear magnetic resonance, and recently, cryo-electron microscopy, can already determine a protein’s structure, they require trial-and-error procedures that can span years in heavy lab work and cost millions in equipment.
However, a major obstacle stood in the race for protein structure prediction technology: a protein can undergo 10^300 different folds before it settles into its final 3D structure. In order to encourage research and development to find a solution to this challenge, Professor John Moult and Krzysztof Fidelis founded CASP in 1994, a biennial contest that assesses participants’ ability to predict protein structure accurately based on experimental data. Accuracy is scored on a metric called the Global Distance Test (GDT), which is the percentage of amino acids within a threshold distance from their correct positions. As Professor Moult stated, a score of 90 GDT is considered to be competitive with experimental results. AlphaFold entered CASP in 2018 and won first place amongst its competitors, but in CASP 2020, AlphaFold rose to the summit of the mountain of decades-old protein structure prediction research and development, outperforming its 100 competitors. In comparison to median scores for best competitors in previous CASP competitions, which ranged from 30 to 40 GDT, AlphaFold managed to obtain a median score of about 56 GDT in CASP 2018 and improved to an 87 GDT in CASP 2020. With such excellent scores in CASP, AlphaFold was deemed a solution to the protein structure prediction challenge by Moult and Fidelis, opening up a pathway for AlphaFold as a primary tool in scientific research.
But how does AlphaFold topple over other AI in making precise protein structure predictions? During its initial development, AlphaFold applied a method known as deep learning, a type of AI function consisting of algorithms closely mimicking the workings of the human brain and neural networks, to predict the distance between pairs of amino acids in a protein and the angles between chemical bonds connecting these amino acids. AlphaFold then searched for a model that fit the protein’s properties. Later on, DeepMind developed and implemented their own new deep learning network, including information about the physical and geometrical constraints of protein folding to upgrade AlphaFold. In its latest version, the folded protein is visualized as a spatial graph, which is used to analyze the physical interactions within proteins. Using an attention-based neural network system, AlphaFold can focus on a subset of inputs, interpret the structure of the graph, and use methods such as evolutionarily related sequences, multiple sequence alignment, and a representation of amino acid pairs to refine the graph. These procedures, alongside a database of about 170 thousand protein structures, is what allows AlphaFold to create highly accurate predictions of protein structures within a few days.
The latest version of AlphaFold has opened numerous possibilities in various fields of biology. With a more efficient and inexpensive way to identify new protein structures and determine their functions, scientists can use AlphaFold for faster drug development as new diseases are being rapidly discovered. “This is a problem that I was beginning to think would not get solved in my lifetime,” stated Janet Thornton, a structural biologist at the European Bioinformatics Institute and a past CASP assessor. Thornton hopes that with AlphaFold, scientists will be able to identify the functions of thousands of proteins and gene variations of these proteins that cause genetic disorders. As for environmental science, AlphaFold can search for enzymes that decompose industrial waste, which may lead to the development of potential solutions for pollution, such as genetically modifying bacteria to synthesize these enzymes.
AlphaFold may even provide future pandemic solutions by providing a more comprehensive understanding of novel viruses by identifying their proteins. DeepMind’s group of researchers have already used AlphaFold to combat the current COVID-19 pandemic by predicting six proteins of the SARS-CoV-2 virus. AlphaFold demonstrated especially accurate predictions for the structure of two SARS-CoV-2 proteins known as ORF3a and OFR8, whose respective functions are promoting cell death and immune system bypassing.
AlphaFold has solved a 50-year-old protein structure prediction problem, and its potential in diverse areas like drug development, industrial waste reduction, and pandemic response not only shows how significant proteins are but what AI is capable of in the life sciences. As one of the first AI networks developed to aid our understanding of how proteins work, AlphaFold will become part of the bridge that branches our knowledge of biology into the new developing field of AI and life-like machines. For Stuyvesant students, this can not only be an exciting new achievement to follow and keep up to date with but also become an inspiration for new interdisciplinary projects and ideas connecting these two fields to expand our scientific expertise. As a more accessible, cheaper means of scientific research, AlphaFold opens up endless opportunities for young STEM students and the global scientific community to continue studying proteins as the essential units of our body and as a future solution to tackle medical, environmental, and various scientific world issues.