Facing a New Reality
Deepfakes are becoming more prevalent in our modern society, but are we ready for the changes they will bring?
Reading Time: 4 minutes
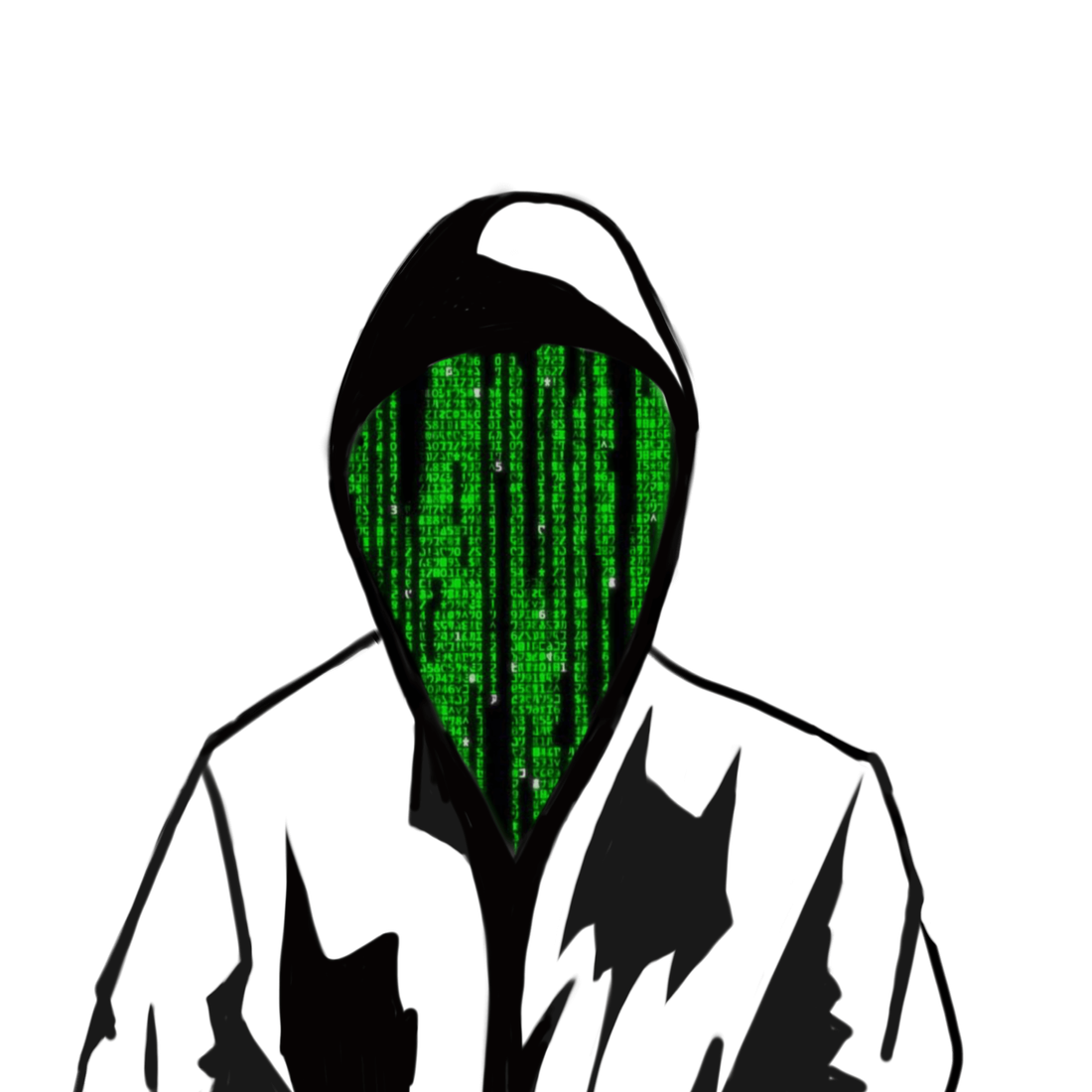
Seeing faces on a screen has become a normal, even trivial part of our lives. Through online classes, social media, and video calls with friends, we reinforce a close association between the pixels on our screens and the people on the other end. Therefore, it may be surprising to hear that these images are not always the product of a person in front of a camera, but rather one of a computer and its algorithms: a deepfake.
A portmanteau of “deep learning” and “fake,” deepfakes can alter a person’s identity in a matter of seconds using artificial intelligence. With deep learning, computer programs can analyze vast sets of data to create a perfect render or animation of a person’s face onto a different body. Social media apps like Snapchat, TikTok, and Instagram use this technology on a face to create entertaining results. A recently released app called WOMBO makes it easier than ever before for users to create deepfake videos. Consequently, there have been videos of Elon Musk and other celebrities lip-syncing to popular songs on social media. Though these videos are evidently parodies and for humor, they reveal the ever-growing power and sophistication of deepfake technology.
WOMBO videos are easy to identify as deepfakes because of their colorful logos, background distortions, and unnatural face movements. On the contrary, more convincing deepfakes remove these small details and fine-tune the facial movements until they appear natural. As a result, the use of deepfake technology demands great responsibility because fake news and misinformation can spread easily through the Internet. Currently, deepfakes aimed at defaming celebrities and spreading misinformation are arousing government attention and public concern. While there are steps to create legislation to ban deepfakes altogether, much of the effort has been focused on creating detection software. Sophisticated deepfake detection is not easy: computers must analyze on the scale of individual pixels to find evidence of tampering. What makes this especially challenging, though, is the alarming rate at which deepfake technology is improving.
Deepfake creation requires generative adversarial networks (GANs), a class of machine learning frameworks that makes two neural networks—a creator and an analyzer—compete against each other. With deepfakes, the creator works to the best of its ability to produce realistic faces, which are then inspected by the analyzer to compare with a large set of actual faces. The deepfake is complete once the analyzer fails to identify the generated face. This process is part of an artificial intelligence (AI) revolution called unsupervised learning, or “AI imagination.” Because of this complex and arduous process, deepfake detection software must overcome this severe, ever-changing challenge.
Currently, the three main ways to detect deepfakes are hand-crafted models, learning-based models, and artifact detection. Hand-crafted models refer to manually detecting imperfections in algorithmic-created facial features. A report published by University of California Berkeley researchers indicates that an individual has distinct, but not unique facial expressions as they speak. Accordingly, the researchers used a software called OpenFace2 to extract facial movements as vectors of facial features from a video, which are then cross analyzed with a deepfake video. By associating numerical values to a source video, this detection software can easily detect irregularities in deepfake videos. In contrast, learning-based models employ neural networks to spot patterns and features of deepfakes. These networks analyze videos for discrepancies in motion that often occur as a result of deepfaking. Artifact detection involves spotting evidence of video manipulation, which includes photo response non-uniformity patterns (PRNU) on images captured by cameras. PRNUs are noise patterns, or disturbances in image brightness and color, that vary depending on the camera model. A lack of PRNUs, or unnatural noise distribution in a video, could be a sign that a GAN, rather than a camera, produced an image or video. If GANs themselves could produce artifact fingerprints of their own, they can be cross analyzed with authentic videos to detect proof of manipulation. Additionally, skin color analysis can reveal blood circulation. Blood circulation patterns, or lack thereof, can then reveal whether or not the subject of a video is a real person or an impostor.
While these innovations are impressive, deepfake manufacturers can circumvent detection in multiple ways. For example, low-quality compressed videos prevent learning-based models from accurately deconstructing deepfakes. Even then, high-quality deepfakes are made to better simulate motion with the use of 3D modeling and more advanced AI. Other deepfakes can overload detection software by simulating too much simultaneous movement in various points of focus. What is more distressing is that GANs can be programmed to remove fingerprint artifacts in an altered video while maintaining the same results. These factors combined make it more difficult to apply detection software to media that may differ greatly from those tested in experiments. This problem becomes more severe when controversial deepfakes spread and are altered more frequently on the internet. When videos are uploaded on different platforms or repeatedly on the same platform, the quality declines based on the uploader’s device and how each platform processes the video. Therefore, if a viral video of a celebrity or political figure gets reposted around the internet, it would become difficult to accurately determine its legitimacy.
Because it is difficult to estimate the limit of AI advancement and deep learning, researchers and computer scientists are still unsure which side will win the war between deepfake creation and detection. Though it is frightening to imagine deepfakes destroying trust in online media, they can also educate people. Schools can use deepfake videos to create a more engaging curriculum with historical figures or world leaders delivering speeches directly to students. For example, the Illinois Holocaust Museum uses deepfake technology to allow visitors to conduct real-time interviews with individuals who have since passed. If used appropriately, deepfakes can also achieve an international impact with notable figures delivering powerful speeches in various languages, as shown with David Beckham delivering multilingual speeches to spread awareness on malaria. Especially now, seeing a full face is a major step toward normalcy; with deepfakes, political leaders can continue to deliver powerful speeches without being present in public and wearing a face mask.
Deepfakes are a byproduct of the unprecedented rate at which current AI technologies like machine learning and neural networks are advancing. Regardless of their negative impact, deepfakes can continue to educate and push researchers to greater heights. The inventor of GANs, Ian Goodfellow, sees a bright future for deep learning and AI: “I hope that AI will help us to develop new medicinal techniques and green energy technologies.” The many faces of deepfakes—entertainment, education, propaganda, and slander—are collectively driving the initiative for AI innovation.