On The Hunt for the Ultimate AI
Despite progress in the field of AI, achieving true artificial general intelligence requires overcoming limitations in human-like reasoning and an understanding of the physical world.
Reading Time: 5 minutes
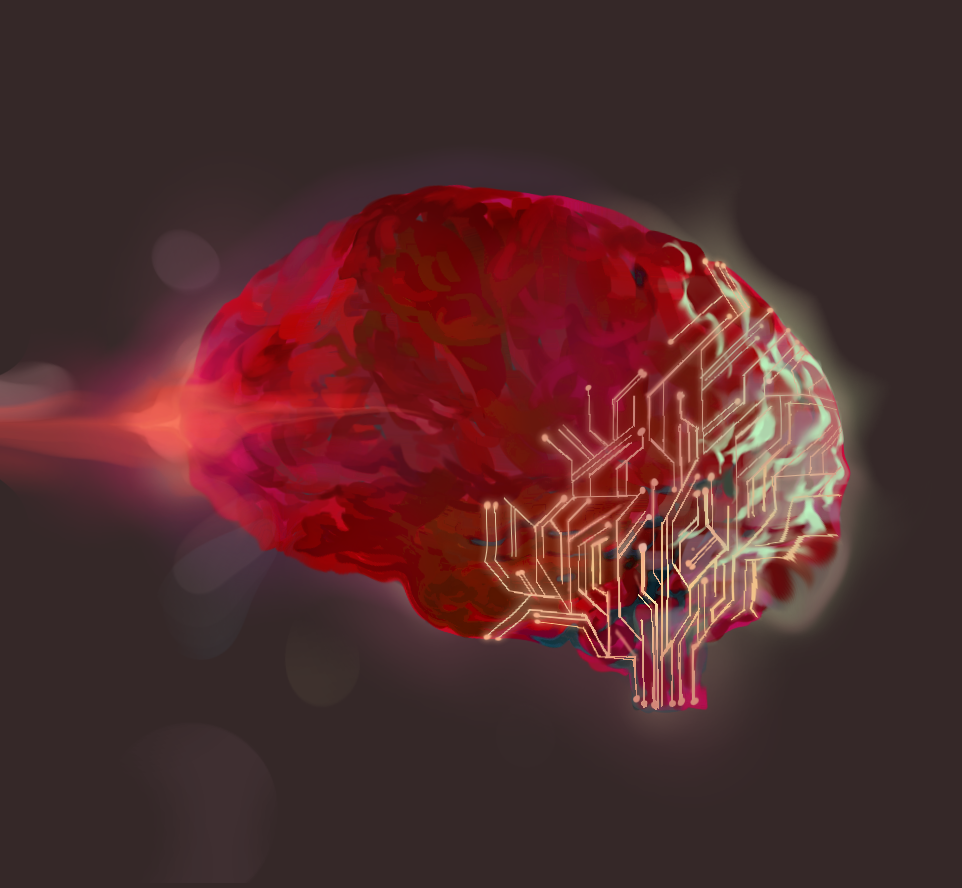
If you’ve ever played around with modern chatbots like ChatGPT, you might have noticed one major flaw—a tendency to give obviously incorrect answers to relatively simple prompts that a human could answer with ease. While basic Artificial Intelligence (AI) models like these have come to impact many aspects of our lives, many AI researchers and major players in the field are hunting a bigger, currently-theoretical target—Artificial General Intelligence (AGI). AGI differs from normal AI in how it would be based on how humans think. It would have more human-like abilities, like logical reasoning, adaptability, and creativity. Current AI models generally try to simulate and imitate training data, but AGI would be able to take it a step further, coming up with new solutions and ideas rather than replicating existing ones.
The term AGI dates back to the late 1980s, but was seen as a synonym for AI, which was also believed to be a sort of human-like intelligence. However, early researchers used a top-down approach to developing AGI in which there would be pre-programmed methods for an AI to achieve a desired outcome. A method more commonly used today is a bottom-up approach in which “common sense” and learning methods are designed first and linked together, which better simulates a human mind.
Current language models, like ChatGPT, can be seen more as machines that guess the next word in a sentence than algorithms that actually understand those words. The latter ability is a critical aspect for a hypothetical AGI. It should be able to have common sense based on an understanding of the physical world, as well as an understanding of causation, allowing it to form complex logical connections. To achieve human-level intellect, it should be able to go above and beyond basic tasks, and apply its knowledge to be able to problem solve in unfamiliar situations. It should also be able to understand the nuances of language, like sarcasm or subtle humor, that would be undetectable to a basic AI language model.
One promising tool to create an AGI is the use of neural networks, due to their ability to learn and improve over time. These networks are based on a human brain and consist of a web of “neurons” stacked in layers, with an input layer, an output layer, and layers in between. Each of these neurons take a particular input and create an output, which it then passes on to the next neuron. They have the ability to learn by giving particular weights to different neurons depending on how much of a role they play in the final result, with the weights determining the influence each neuron has on the final output. This ability to adapt to recognize patterns and tasks would allow an AGI to adapt to new information that it receives.
Building on the capacities of neural networks, deep learning involves training neural networks to extract relationships based on large amounts of data fed into them. Deep learning’s pattern recognition and ability to learn from examples when given a large dataset give it the potential to be applied to AGI. However, one weakness of deep learning is its inability to generalize its knowledge to objects or patterns that it hasn’t encountered before. This is a critical challenge faced by developers, as the data used to train an AI can’t always reflect the nuances and complexities faced by an AGI in the real world. In deep learning, models create rules and attempt to fit everything they encounter into those rules, while a human or an ideal AGI would assess a situation as it encounters it even if it hasn't encountered something similar before.
One issue with current AI models is their opacity. Humans can find it difficult to understand the decisions made by current AI models, which isn’t ideal for an AI that should have a clear line of reasoning for its decisions and outputs. Explainable AI, or XAI, is a field of research to understand how AI makes decisions based on their training data. This would allow humans to identify potential biases, logical flaws, or other weaknesses in the decision-making process, allowing AI models to be more trustworthy and explainable. Understanding the weaknesses of AI models would allow humans to enhance the capabilities of an AGI and ensure its reasoning and logic is sound.
There are a number of potential ways for users to interact with an AGI, as well as ways that an AGI could interact with the world. Currently, the general public uses AI models through websites or apps on their devices to generate text or videos. However, new technologies like virtual reality or augmented reality could also be used, or chips like Neuralink that are physically implanted into the body. Robots also serve as a promising platform, as they allow AGI to interact with the physical world. This interaction with physical objects is also crucial, as it allows an AGI to understand the world. Through physically touching and feeling objects, an AGI will learn critical aspects about the physical world, like the fact that a stove is hot or an egg is fragile.
The ability to intuit and truly “think” is the primary hurdle for the development of AGI, as current AI models are heavily dependent on the data that they are trained upon and fail to make genuine connections. Additionally, the inability to physically interact with the world like humans do is a significant obstacle when it comes to understanding the world. Creativity and emotional intelligence require an AGI to be able to read between the lines to understand the tones of speech, which current models are unable to do. Additionally, developers must work to mitigate biases in an AGI and be able to control it without impeding its abilities. The latter is particularly challenging, as an autonomous AGI without human oversight would have to have built-in limitations in regard to the information it can access or the actions it can take, which would reduce its capabilities.
Controversy exists over the possible timelines for the development of AGI. While many scientists believe AGI will appear by the 2060s, some have argued that newer versions of current language models, like GPT-4, have already shown potential of being much closer to AGI than their predecessors. But one thing is clear—there will be no single solution or approach to achieving a fully functional AGI, and many different aspects of current AI research must be combined to simulate the complexity of the human brain. Advances in sensor technology and robotics must be made to allow an AGI to interact with and sense the world the way humans do, giving them improved cognition. Additionally, computing advances will also be necessary to handle the massive amounts of data required to train new AI models. The future for AGI is promising, but we must ensure that, in the end, it is used responsibly and for the benefit of humanity.